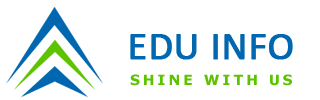
Intelligent electrocardiogram pattern classification and recognition using low-cost cardio-care system Electrocardiogram (ECG) contains detailed information regarding incidental abnormality of a subject. Manual analysis of a long time ECG record is a lengthy process. Computerised ECG analysis supports clinicians in decision making. While designing a low-cost diagnostic support system, constraints on the system resources limit the processing speed, eventually affecting the reliability. To resolve these issues, three key factors have been addressed in this study: the feature extraction method, total number of features and the database used. For feature extraction, `polar Teager energy’ algorithm has been developed, yielding nearly 70% saving in processing time as compared to other well-known methods. Using features with linear relationship leads to reduction in feature vector dimension, without compromising its classification performance. Therefore the linear relationship between two ECG features, namely `informational entropy'(S) and `mean Teager energy’ has been revealed. These features are utilised for ECG beat classification using `fuzzy C-means clustering’ algorithm. The algorithm is evaluated using the MIT-BIH database and then tested by ECG measured with the cardio-care unit. The QRS detection performance of the proposed method is very good, with 0.27% detection error rate. For classification of ECG beats, average sensitivity and positive prediction rate achieved are 98.93% each.