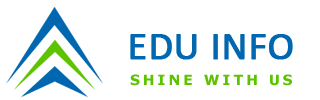
SMapReduce: Optimising Resource Allocation by Managing Working Slots at Runtime Hadoop version 1 (HadoopV1) and version 2 (YARN) manage the resources in a distributed system in different ways. HadoopV1 executes MapReduce tasks in working slots that are statically configured, YARN uses a set of task containers to encapsulate its memory and CPU resources. However, neither of them considers the runtime performance of the cluster when deciding the proper number of concurrent tasks to run on each node to achieve the optimal throughput. In order to gain higher performance, the users of Hadoop usually need to use their experience to carefully configure the resources of the cluster and the resources needed by their jobs. But as the workload is typically always changing in the cluster, rarely could such a manual configuration lead to optimized performance. In this paper, we study the MapReduce job performance in HadoopV1 and YARN with different resource configurations, and model the cluster throughput in terms of the resource capacity of the cluster. We propose SMapReduce, which can dynamically manage a proper number of concurrent tasks running on each node. SMapReduce can gain the maximum job throughput by considering the thrashing phenomenon and the balancing between map and reduce tasks. Evaluation results show that SMapReduce can yield significant performance speedup comparing to both HadoopV1 and YARN for various MapReduce workloads.