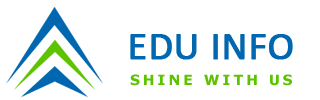
Hybrid dynamic data mining scheme for drift-like fault diagnosis in multicellular converters This paper proposes a data-mining based scheme in order to build a classifier able to achieve a reliable drift monitoring in normal operating conditions of multicellular converters (MCC). The goal is to achieve an early diagnosis of faults that can affect the behavior of MCC. This scheme considers the converter as a discretely controlled continuous system. Therefore, it takes into account the converter continuous dynamics in each discrete mode. This allows obtaining a feature space sensitive to normal operating conditions in each discrete mode.