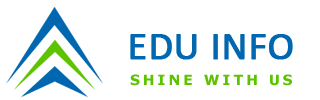
Accelerated PSO Swarm Search Feature Selection for Data Stream Mining Big Data Big Data though it is a hype up-springing many technical challenges that confront both academic research communities and commercial IT deployment, the root sources of Big Data are founded on datastreams and the curse of dimensionality. It is generally known that data which are sourced from datastreams accumulate continuously making traditional batch-based model induction algorithms infeasible for real-time data mining. Feature selection has been popularly used to lighten the processing load in inducing a data mining model. However, when it comes to mining over high dimensional data the search space from which an optimal feature subset is derived grows exponentially in size, leading to an intractable demand in computation. In order to tackle this problem which is mainly based on the high-dimensionality and streaming format of data feeds in Big Data, a novel lightweight feature selection is proposed. The feature selection is designed particularly for mining streaming data on the fly, by using accelerated particle swarm optimization (APSO) type of swarm search that achieves enhanced analytical accuracy within reasonable processing time. In this paper, a collection of Big Data with exceptionally large degree of dimensionality are put under test of our new feature selection algorithm for performance evaluation.