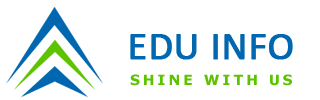
COPAL — Cognitive personalized aid for learning Concept learning can be illustrated based on a layered model of knowledge discovery. To discover association between learners’ requirements and learning materials we propose a cognitive learning architecture. Development of cognitive personalized aid for learning (COPAL) involves utilizing the concepts of cognitive informatics for providing peer interaction between learner and system and to deal with the challenge of handling unstructured Big E-learning data. Cognitive learning system utilizes the concept of data mining and natural language processing. We present a learner centered strategy of learning using open source Hadoop cluster. The paper describes development of system in which the cognitive learning engine interacts with the learner and the personalized recommender to satisfy the learner needs. Our system provides a scalable collaborative framework for constructing recommendations using extensible library of data mining provided by Apache Mahout. HDFS has been employed for reliability, scalability and low cost storage capability. During data preprocessing Pig has been used to transform unstructured Big E-learning data. The paper demonstrates the use of Pearson correlation and Euclidean distance for similarity computation. Our implementation found it beneficial to use Euclidean similarity metric. It is thus feasible to develop efficient E-learning recommender system coupled with cognitive framework using open source software.