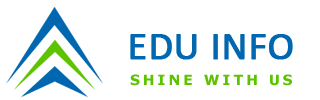
Discretization of Simulated Flight Parameters for Estimation of Situational Awareness Using Dynamic Bayesian Networks In the context of a PhD thesis on data mining, we have implemented a simulation environment that collects data for measurements of certain aspects of the Situational Awareness (SA) of a pilot using Bayesian networks (BN). The tool is based on a web application that emulates an Electronic Flight Bag (EFB) and is connected to a flight simulator, providing the user with basic autopilot controls and a customizable interface to access aeronautical information. Relevant data concerning to actions of the pilot, information queries and flight parameters are stored in a database. The use of System Wide Information Management (SWIM) technologies is specially applicable to this research because they provide a robust and powerful approach to the exploration of data relationships. But before analyzing the probabilistic dependencies of the dataset collected during the simulation, it is necessary to study how variables are adapted to the requirements of a Dynamic BN (DBN). This paper briefly presents some SA rating techniques and our approach to achieve a relevant measurement that relies on cockpit information management and DBN, focusing on the first experiment performed with the simulation environment, that analyzes the influence of different discretization criteria on the scores obtained by DBN that learn variable dependencies from data.