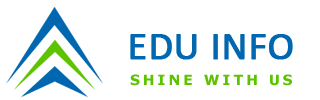
Exploring concept graphs for biomedical literature mining Full-text publications in an electronic form become more prevalent than ever before. It is a difficult challenge to extract concepts from unstructured document collections data because different concepts and their relationships are buried in them and ample term variations make the challenge compound. Extracted concepts are useful instruments of managing and searching large document collections and play a pivotal role in indexing electronic documents and building digital libraries. In this paper we explore a biomedical concept extraction technique based on a ranking algorithm of concept graphs. The proposed technique comprises two major steps: the first step is to represent documents with graphs whose nodes and edges are created by Named Entity Recognition and UMLS Semantic Network. The second step is rank concepts with relative importance algorithms. We evaluate our technique with a set of biomedical full-texts and compare it to various different key-phrase extraction and graph ranking techniques. The experimental results show that our technique achieves the best performance over other compared algorithms. We further take a close look at the properties of the network to examine how concepts are related to each other and what concept plays a dominant role in the network. To this end, we build the network with 526 full-text articles published in PubMed Central and measure the significance of nodes by centrality.