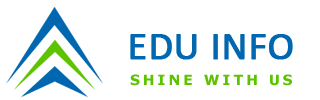
On Resolving Simultaneous Congruences Using Belief Propagation Graphical models and related algorithmic tools such as belief propagation have proven to be useful tools in (approximately) solving combinatorial optimization problems across many application domains. A particularly combinatorially challenging problem is that of determining solutions to a set of simultaneous congruences. Specifically, a continuous source is encoded into multiple residues with respect to distinct moduli, and the goal is to recover the source efficiently from noisy measurements of these residues. This problem is of interest in multiple disciplines, including neural codes, decentralized compression in sensor networks, and distributed consensus in information and social networks. This letter reformulates the recovery problem as an optimization over binary latent variables. Then we present a belief propagation algorithm, a layered variant of affinity propagation, to solve the problem. The underlying encoding structure of multiple congruences naturally results in a layered graphical model for the problem, over which the algorithms are deployed, resulting in a layered affinity propagation (LAP) solution. First, the convergence of LAP to an approximation of the maximum likelihood (ML) estimate is shown. Second, numerical simulations show that LAP converges within a few iterations and that the mean square error of LAP approaches that of the ML estimation at high signal-to-noise ratios.