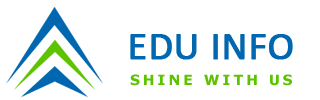
Real-Time Analytics for Fast Evolving Social Graphs Existing Big Data streams coming from social and other connected sensor networks exhibit intrinsic inter-dependency enabling unique challenges to scalable graph analytics. Data from these graphs is usually collected in different geographically located data servers making it suitable for distributed processing on clouds. While numerous solutions for large scale static graph analysis have been proposed, addressing in real-time the dynamics of social interactions requires novel approaches that leverage incremental stream processing and graph analytics on elastic clouds. We propose a scalable solution based on our stream processing engine, Floe, on top of which we perform real-time data processing and graph updates to enable low latency graph analytics on large evolving social networks. We demonstrate the platform on a large Twitter data set by performing several fast graph and non-graph analytics to extract in real-time the top k influential nodes, with different metrics, during key events such as the US NFL playoffs. This information allows advertisers to maximize their exposure to the public by always targeting the continuously changing set of most influential nodes. Its applicability spans multiple domains including surveillance, counter-terrorism, or disease spread monitoring. The evaluation will be performed on a combination our local cluster of 16 eight-core nodes running Eucalyptus fabric and 100s of virtual machines on the Amazon AWS public cloud. We will showcase the low latency in detecting changes in the graph under variable data streams, and also the efficiency of the platform to utilize resources and to elastically scale to meet demand.