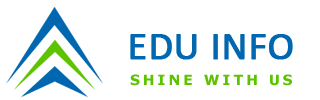
Dataless Data Mining: Association Rules-Based Distributed Privacy-Preserving Data Mining Today, the desire to mine data from varied sources to discover behaviors and patterns of entities such as customers, diseases, and environmental conditions is on the rise. At the same time, the resistance to share data is also on the raise due to the increase in governmental regulations and individuals desire to preserve privacy. In this paper, we employ association rule mining to preserve individual data privacy without overly compromising on the accuracy of the global data mining task. Here, we describe the proposed methodology and show that the proposed scheme is privacy preserving. The methodology is tested using three commonly available data sets. The results validate our claims regarding the accuracy of synthetic data in its ability to represent original data without compromising privacy.