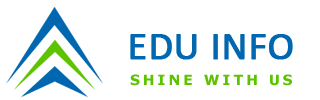
Extended Kalman Filter-Based Parallel Dynamic State Estimation There is a growing need for accurate and efficient real-time state estimation with increasing complexity, interconnection, and insertion of new devices in power systems. In this paper, a massively parallel dynamic state estimator is developed on a graphic processing unit (GPU), which is especially designed for processing large data sets. Within the massively parallel framework, a lateral two-level dynamic state estimator is proposed based on the extended Kalman filter method, utilizing both supervisory control and data acquisition, and phasor measurement unit (PMU) measurements. The measurements at the buses without PMU installations are predicted using previous data. The results of the GPU-based dynamic state estimator are compared with a multithread CPU-based code. Moreover, the effects of direct and iterative linear solvers on the state estimation algorithm are investigated. The simulation results show a total speed-up of up to 15 times for a 4992-bus system.