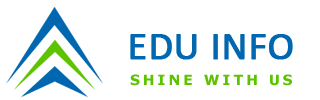
Use of rule base data mining algorithm for intrusion detection Due increased growth of Internet, number of network attacks has been increased. Which emphasis need for intrusion detection systems(IDS) for secureing network. In this process network traffic is analyzed and monitored for detecting security flaws. Many researchers working on number of data mining techniques for developing an intrusion detection system. For detecting the intrusion, the network traffic can be classified into normal and anomalous. In this paper we have evaluated five rule base classification algorithms namely Decision Table, JRip, OneR, PART, and ZeroR. The comparison of these rule based classification algorithms is presented in this paper based upon their performance metrics using WEKA tools and KDD-CUP dataset to find out the best suitable algorithm available. The classification performance is evaluated using crossvalidation and test dataset. Considering overall higher correct and lower false attack detection PART classifier performs better than other classifiers.